By Linda Kreitzman, Executive Director of the Master of Financial Engineering Program at Berkeley
Master of Financial Engineering programs’ students have, in my view, a very wide array of job opportunities in front of them, and not just in traditional quantitative finance with roles as strats at an I-bank, or researchers/portfolio managers at a hedge fund, derivatives traders, or in high frequency trading, etc. Financial disintermediation from Fintech firms brought opportunities in areas such as digital payments, robo-advising, peer-to-peer lending, digital insurance (InsurTech), healthcare, etc. We can safely say that domains of all financial intermediation have been impacted by digital finance and that they created data science roles for financial engineers. Additionally, with the decentralization of finance (DeFi/blockchain/space), there are more and more opportunities as data scientists at crypto brokerages/exchanges/funds/VCs (examples: Coinbase, Pantera Capital, Coinfund, BlocKFi, etc.) and as crypto traders, and not just developer roles. On this front, expect more trading opportunities in DeFi coming from investment banks and hedge funds too, as they are now considering cryptocurrencies as a new asset class which should be part of a diversified portfolio.
Personally, for many years now, I have seen more and more students coming out of financial engineering programs pursue data scientist roles because they wanted to use their AI, data, and machine learning skill set. In fact, in 2012, one of our graduates was hired by Facebook right after graduation as the first data scientist in our program. He went on to work for Instagram where he led the analytics team, then Twitter where he grew the data science team within the engineering team, and, after Twitter, he founded his own Fintech in healthcare.
After 2013-14, more tech or fintech firms began to have a strong interest in quantitative students and vice-versa: Google, Uber, Lending Club, Amazon, OpenDoor, Twitch, Unison, Square, Stripe, Wealthfront, Betterment, etc., hired more and more data scientists among financial engineering students because of their strong computer science, data structures, math, statistics, machine learning modeling, deep learning, and finance skills. And while quant finance experienced a huge boom till the 2008 crisis, gradually, students became less enamored with a traditional job in quant finance using stochastic calculus, C++, backtesting, etc. They began to consider roles as data scientists because they found it a lot more interesting to work with structured and unstructured data to find solutions to complex problems faced by organizations.
To understand better why a graduate would choose a data science role over one in “pure finance”, I would like to give Quantnet readers the example of one of our alumni who had the choice between working at a big asset management firm in San Francisco and Google after graduation. A good friend of his with whom he had worked with in finance prior to the MFE asked him to join her team at Google. He took the leap to join Google because he had enjoyed working with her before. Interestingly, he chose to join the Ads Marketing team as a data scientist over work on ETFs. His responsibility was to determine which actions the marketers performed were valuable to Google. This involved A/B testing and statistics around email campaigns, events, education, and brand marketing. He would then build models or analyses to help determine what actions the team should do next or how to improve the strategy going forward.
Everything he learned in all the MFE including the Derivatives course on how to approach problems with the correct technical rigor and Monte Carlo simulation as well as courses on linear and logistic regression helped him tremendously. He is now at Waymo, developing self-driving cars and helping software engineers determine if the changes they make improve the vehicle's performance.
At Waymo, the data science team answers two main questions: How did we do and what should we do next? The first question involves statistics of A/B testing and time series analysis. The second question involves segmentation, Monte Carlo simulations, linear/logistic regression and other types of machine learning models like tree-based models. These involve building tools and models in SQL, Python, and other languages too.
One may wonder “how close to finance" the role of data scientist is. Our alumnus at Waymo would argue that the skill sets and technical rigor are similar, just applied to different domains. When I asked him, he replied, “We have to make sense of data and apply it to something useful which can be self-driving cars or valuing derivatives. We then have to use that to make decisions about the future (Ex. should we push these changes to the car or is this the appropriate level of risk in the portfolio?) I use a majority of the skills that I learned in the MFE simply applied to the self-driving car context.”
If you’d ask him if the MFE was the right field of study to pursue, our MFE17 would say yes, of course. Like him and all data scientists “produced” by our MFE program, they would not have been able to perform or even attempt their roles without the MFE. The MFE teaches one how to approach problems in a technical and rigorous way and the internship gives all a strong foundation of projects to discuss in interviews showing the application of those methods.
And of course, one may also wonder whether data scientists coming from FE programs “miss finance,” but they invariably answer that they do not. They are excited about the problems they are working on, they love the culture at their firms, the (better) work-life balance, and the free food, well, except during the shelter-in-place! Of course, they still keep track of the financial markets.
Ultimately, the roles and use of skills learned in FE programs are comparable applied to different domains. And as a data scientist, you can always move back (or move) to a role in quantitative finance or vice versa.
Just make sure you consider all roles available to financial engineers and they are plenty to choose from! I can say without a doubt that, at this time, the sky's the limit- literally. Data scientists, machine learning engineers (same role as data scientists for many firms), product and data engineer or analyst/associate, etc., and, of course, all traditional quant finance roles abound too at this time.
Know your skillset, what you want to do and always keep track of the innovation in finance because DeFi is another big opportunity to pay attention to, even if regulation is on "its way."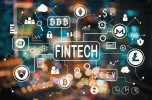
Personally, for many years now, I have seen more and more students coming out of financial engineering programs pursue data scientist roles because they wanted to use their AI, data, and machine learning skill set. In fact, in 2012, one of our graduates was hired by Facebook right after graduation as the first data scientist in our program. He went on to work for Instagram where he led the analytics team, then Twitter where he grew the data science team within the engineering team, and, after Twitter, he founded his own Fintech in healthcare.
After 2013-14, more tech or fintech firms began to have a strong interest in quantitative students and vice-versa: Google, Uber, Lending Club, Amazon, OpenDoor, Twitch, Unison, Square, Stripe, Wealthfront, Betterment, etc., hired more and more data scientists among financial engineering students because of their strong computer science, data structures, math, statistics, machine learning modeling, deep learning, and finance skills. And while quant finance experienced a huge boom till the 2008 crisis, gradually, students became less enamored with a traditional job in quant finance using stochastic calculus, C++, backtesting, etc. They began to consider roles as data scientists because they found it a lot more interesting to work with structured and unstructured data to find solutions to complex problems faced by organizations.
To understand better why a graduate would choose a data science role over one in “pure finance”, I would like to give Quantnet readers the example of one of our alumni who had the choice between working at a big asset management firm in San Francisco and Google after graduation. A good friend of his with whom he had worked with in finance prior to the MFE asked him to join her team at Google. He took the leap to join Google because he had enjoyed working with her before. Interestingly, he chose to join the Ads Marketing team as a data scientist over work on ETFs. His responsibility was to determine which actions the marketers performed were valuable to Google. This involved A/B testing and statistics around email campaigns, events, education, and brand marketing. He would then build models or analyses to help determine what actions the team should do next or how to improve the strategy going forward.
Everything he learned in all the MFE including the Derivatives course on how to approach problems with the correct technical rigor and Monte Carlo simulation as well as courses on linear and logistic regression helped him tremendously. He is now at Waymo, developing self-driving cars and helping software engineers determine if the changes they make improve the vehicle's performance.
At Waymo, the data science team answers two main questions: How did we do and what should we do next? The first question involves statistics of A/B testing and time series analysis. The second question involves segmentation, Monte Carlo simulations, linear/logistic regression and other types of machine learning models like tree-based models. These involve building tools and models in SQL, Python, and other languages too.
One may wonder “how close to finance" the role of data scientist is. Our alumnus at Waymo would argue that the skill sets and technical rigor are similar, just applied to different domains. When I asked him, he replied, “We have to make sense of data and apply it to something useful which can be self-driving cars or valuing derivatives. We then have to use that to make decisions about the future (Ex. should we push these changes to the car or is this the appropriate level of risk in the portfolio?) I use a majority of the skills that I learned in the MFE simply applied to the self-driving car context.”
If you’d ask him if the MFE was the right field of study to pursue, our MFE17 would say yes, of course. Like him and all data scientists “produced” by our MFE program, they would not have been able to perform or even attempt their roles without the MFE. The MFE teaches one how to approach problems in a technical and rigorous way and the internship gives all a strong foundation of projects to discuss in interviews showing the application of those methods.
And of course, one may also wonder whether data scientists coming from FE programs “miss finance,” but they invariably answer that they do not. They are excited about the problems they are working on, they love the culture at their firms, the (better) work-life balance, and the free food, well, except during the shelter-in-place! Of course, they still keep track of the financial markets.
Ultimately, the roles and use of skills learned in FE programs are comparable applied to different domains. And as a data scientist, you can always move back (or move) to a role in quantitative finance or vice versa.
Just make sure you consider all roles available to financial engineers and they are plenty to choose from! I can say without a doubt that, at this time, the sky's the limit- literally. Data scientists, machine learning engineers (same role as data scientists for many firms), product and data engineer or analyst/associate, etc., and, of course, all traditional quant finance roles abound too at this time.
Know your skillset, what you want to do and always keep track of the innovation in finance because DeFi is another big opportunity to pay attention to, even if regulation is on "its way."
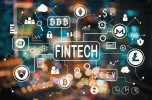